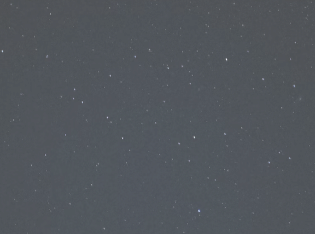
Caylent Catalysts™
Serverless Data Lake
Rapidly implement a foundational low-code data lake with Caylent's data engineering experts who will also enable your teams for no-code exploratory data analysis.
Caylent Catalysts™
Rapidly implement a foundational low-code data lake with Caylent's data engineering experts who will also enable your teams for no-code exploratory data analysis.
Caylent Catalysts™
From implementing data lakes & migrating off commercial databases to optimizing data flows between systems, turn your data into insights with AWS cloud native data services.
Caylent Catalysts™
Accelerate the adoption of a production ready AWS foundation, and establish automated security guardrails to keep existing and new accounts in compliance with your desired security posture.
Plan and implement an MLOps strategy unique to your team's needs, capabilities, and current state, unlocking the next steps in tactical execution by offloading the infrastructure, data, operations, and automation work from data scientists.
The practice of MLOps recognizes the advancements that have been achieved in application development by aligning developers and operations teams (DevOps). It also acknowledges the reality that model engineering is one component within a complex ecosystem that is required to successfully apply machine learning to achieving business objectives.
Caylent's expertise with DevOps, data engineering, machine learning, and production operations combine to provide the multi-disciplinary breadth and depth necessary to successfully plan your MLOps strategy and help implement any or all of the individual components.
While MLOps has proven its worth for early adopters, experienced practitioners are still rare and difficult to attract. Creating a strategy based on our experience and unique to your team's needs, capabilities, and current state unlocks the next steps in tactical execution by offloading the infrastructure, data, operations, and automation work from data scientists.
Through a series of discovery workshops, we’ll review your current processes, technology landscape, and industry best practices for data engineering, model engineering, and runtime operations.
Based on your input, we’ll design data and process flows as well as architecture and implementation plans for infrastructure, data lake, feature store, data pipelines, analysis tools, model development environments, and monitoring.
Following our design collaboration, we'll summarize your data landscape, identify the top priority business cases to address, and craft an implementation plan to begin adopting MLOps.
MLOps Strategy