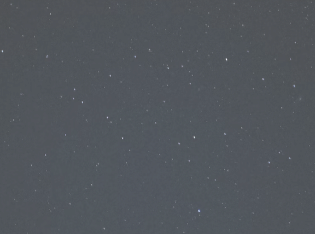
Caylent Catalysts™
AWS Generative AI Proof of Value
Accelerate investment and mitigate risk when developing generative AI solutions.
Learn about all the generative AI industry trends we've seen in 2023, and how these will impact your technology and business landscape.
2023 has been an exceptional year for Generative AI, with AWS making over 40 announcements of various advancements in this space. This blog captures these announcements and presents them in the context of Generative AI industry trends we’ve seen here at Caylent. This blog also offers our perspective on how these trends impact our will impact our customers.
2023 has undoubtedly been a Generative AI proof-of-concept year, with Retrieval Augment Generation (RAG) leading model customization methods. As the market matured, with many organizations realizing RAG’s limitations, the demand for more sophisticated model customization methods that can overcome these limitations, such as fine-tuning and domain adaptation, is rising. These methods will become a central ask in Generative AI solutions, placing a high demand on model customization skills and their relevant services and features. This trend has also emphasized the importance of customer data maturity, requiring large quantities of clean data for model customization.
2023 saw a boom in the number of open-sourced and closed-sourced models entering the market. For example, Hugging Face-hosted models increased by over 16-fold since last year1. This trajectory will continue with more Generative AI models entering the mainstream, contributing to decision fatigue and creating demand for model selection skills.
Models entering the market compete in performance. Model creators increase model sizes and training datasets, specialize in specific tasks, and innovate new model architectures and training procedures to outperform the state-of-the-art. Consequently, these models will become more powerful, performing a more comprehensive range of tasks better than before, making them even more popular.
To date, the power of these models has been closely coupled with their sizes. Model sizes have grown exponentially, with some models reaching the trillion-parameter level, such as Google’s Switch Transformers and the anticipated Amazon Olympus model. The growth of these models' power and size creates demand for more powerful computing and storage options to keep up with model training, hosting, and inference speed requirements. This reality will remain so until we see a scientific breakthrough in decoupling model size and performance for good. Thus, we anticipate significant investments in computing and storage to increase production and alleviate supply chain bottlenecks and limitations. On the other hand, we will see consistent attempts at making models smaller yet more powerful, allowing these models to be used without internet connectivity and deployed on edge devices, such as smartwatches and phones.
Since the start of 2023, we have seen Generative AI getting into the fabric of our everyday professional and personal lives. Many popular applications are offering Generative AI features while new Generative AI-powered applications are entering the market, and countless custom solutions and use cases are being built in all verticals and industries, augmenting processes and workforce and bringing forth a massive economic growth. Generative AI has been truly disruptive.
The adoption of Generative AI and its economic promise are still in their infancy. Generative AI’s clientele will continue to grow, and we will witness more innovative ways of incorporating its power into professional and personal lives. We will continue to see more applications using Generative AI and more roles and functions augmented with Generative AI, resulting in increased demand for Generative AI literacy and application development.
As its popularity increases, Generative AI will create a demand for improving backend operations and integrating supporting services with each other and third-party systems to support advanced use cases’ development, rapid development requirements, and maintainability and operations aspects of Generative AI applications and solutions. This demand will lead to advancements in Generative AI-supporting services, including data processing, storage, retrieval, and governance; Machine Learning Operations for Large Language Models (LLMOps) and model monitoring; and Generative AI tooling.
Generative AI’s unprecedented power has raised concerns regarding its impact on job loss and the democratic process, among other concerns, resulting in calls for responsible AI, security, and regulation. In March this year, over 1000 technology leaders called for a pause in training more powerful AI models. Later this year, the United States President, Joe Biden, signed an executive order introducing stringent compliance and regulatory requirements around AI safety, security, and privacy, and most recently, the UK and 17 other countries signed a non-binding agreement vowing to protect the public from AI misuse. As these models become more powerful and ubiquitous, serious considerations will be paid to their safety, security, and privacy, resulting in attempts to regulate them, leading to advancements in security and model governance, and placing a demand for more features and skill sets in this space.
The recent AWS announcements align seamlessly with these industry trends. From introducing new services and models to advancements in backend technologies and compute resources, AWS is at the forefront of addressing these emerging needs and shaping the future of cloud computing. We delve into these announcements below.
Organizations are increasingly seeking market differentiation by capitalizing on the transformative power of using Generative AI with their data. The understanding that model customization is the vehicle to realizing this power has become common.
Retrieval Augmented Generation (RAG) has been the standard method for model customization in 2023 and will continue to be the standard in the foreseeable future. However, with the limited model context window size, the need for improving these sizes and alternative solutions is quickly rising.
Realizing this, Amazon Web Services (AWS) has made several important announcements to alleviate the context window size limitation to some degree and, more importantly, introduce a host of model customization options.
During re:Invent 2023, AWS announced Anthropic Claude 2.1’s availability in Amazon Bedrock. This Claude model provides a 200 thousand token context window size roughly equivalent to 150 thousand words, doubling the previous version's size. AWS also announced the general availability of Amazon Bedrock’s Fine Tuning, which enables customers to fine-tune many models, including ones from AWS, Meta, and Cohere, on customer data for specific tasks, such as sentence completion. Anthropic’s Claude cannot yet be fine-tuned using this feature, but it is promised to come soon. Meanwhile, customers can work with AWS to customize Anthropic’s model via the newly announced AWS Generative AI Innovation Center’s Custom Model Program for Anthropic Claude. AWS also announced the availability of a continued pre-training feature in Amazon Bedrock, enabling customers to deal with highly volatile data and ensuring their models' relevancy and domain specificity. They also introduced Titan Text Lite, a small, cost-effective new model ideal for customization.
Catering to more technical customers, AWS also announced Amazon SageMaker HyperPod, a distributed training solution for LLMs, which reduces training time and deals with hardware failures during long-running training jobs via an innovative checkpointing feature. The latter capability is significant for LLM training jobs, which take days or weeks and involve hundreds of machines. Hardware failures at this scale can seriously affect a training job's success rate.
More and more customers need help navigating the model choice problem amidst the model plethora we have today. This complex problem requires an adequate understanding of business problems and concerns and strong technical capabilities to understand the nuanced model differences, benchmarking techniques, cost models, and model safety to determine the correct model choice for a particular use case.
The good news is that AWS has been curating cutting-edge models on its platform, distilling them into a subset of high-performing models that cover all common use cases, reducing the model selection burden. Starting with a limited collection earlier this year, AWS announced a series of strategic partnerships, bringing industry-leading models to AWS customers through Amazon Bedrock and SageMaker JumpStart, including:
AWS also expanded their Titan-family models to include new ones with highly performant capabilities, including Titan Image Generator and Amazon Titan Multimodal Embeddings on Bedrock. AWS also curated a set of popular models from TensorFlow Hub, PyTorch Hub, Hugging Face, and Gluon CV and offered them on JumpStart.
Another great announcement this year is regarding model evaluation and benchmarking. Starting with Amazon SageMaker, AWS announced this year that SageMaker Clarify now supports automatic and human-in-the-loop evaluation of foundation models. SageMaker Clarify is not the only service getting this feature. Similarly, AWS announced that Amazon Bedrock now has the functionality to natively evaluate models using pre-defined metrics for specific tasks such as content summarization, question answering, text classification, and text generation, and a native method for human evaluation with a customer-managed team or an AWS-managed team, reducing the burden of designing, running and managing model evaluation benchmarks and workflows.
Generative AI has stormed into the mainstream and started to get into the fabric of our lives, giving people superhuman skills that previously required hundreds of learning hours and practice, such as coding, content generation, and image manipulation. We are witnessing an increase in these models, competing in performance and task coverage. As their creators work on making them more capable, their clientele is increasing and will only continue to grow, becoming subject to consumer expectations and yearly upgrade cycles.
We believe that Generative AI capabilities will continue growing atop what we’ve already seen. Models will get even more powerful and become capable of accomplishing a more comprehensive range of tasks, giving rise to the need for scientific advancements in this area, including model architecture and training. This exciting trend will lead to augmenting or automating more use cases and enhanced performance in those already augmented or automated today, translating to more economic and performance transformations for customers.
One promising advancement is in multimodal models, showing exceptional performances. AWS announced Amazon Titan Multimodal Embeddings, a multimodal model capable of generating embeddings from images and their textual descriptions, empowering a wide range of tasks, such as image-to-image and text-to-image search, image classification, object detection and recognition, image search, retrieval and similarity, and video analysis. This model has wide business applications, such as Content Recommendation and Personalization Systems, Security and Surveillance, Quality Control and Inspection, Medical Imaging and Disease Detection, and Automated Content Moderation.
Furthermore, Amazon Bedrock’s latest model additions, Meta’s LLama 2 and Anthropic’s Claude 2.1, also broke the boundaries of “what is possible”. Claude 2.1 featured a massive improvement in the context window size limitation with its 200,000-token context window size and 50 percent reduced hallucinations compared to its predecessor, among other advancements.
Model providers compete in making models more powerful, producing massive models, such as Amazon’s Olympus, which has been reported to have 2 trillion parameters. How much larger will these models get before they become intractably large, costly to host, and slow to use?
Computing, storage, and model sizes are the Generative AI limitation trifecta in 2023. Generative AI models are large, requiring tremendous computing power to train and query (inference) and large storages with high data access speeds for model training. This trifecta is a barrier facing Generative AI adoption, preventing its ubiquity in everyday applications and devices, such as smartwatches, without an internet connection. Models must shrink without affecting their capabilities, or computing and storage must catch up.
AWS has been at the forefront of dealing with this trifecta by offering cutting-edge computing instances, high-performance storage, and small yet performant models.
In its latest collaboration, in a series of collaborations, AWS signed a strategic collaboration agreement with NVIDIA later this year to bring in NVIDIA’s latest superchip, the GH200 NVL32, also known as the GH200 Grace Hopper Superchip, into AWS EC2 instances, in a strong step in tackling the computing limitation problem. This superchip can provide up to 20 TB of shared memory to power terabyte-scale workloads. AWS has already introduced many computing instances powered by NVIDIA’s Superchips, including Amazon EC2 P5 Instances, Powered by NVIDIA H100 Tensor Core GPUs for Accelerating Generative AI and HPC Applications. AWS Also announced a series of new EC2 instances powered by NVIDIA’s Superchips, including EC2 G6 and EC2 G6e instances.
AWS also announced a series of new instances optimized for various ends, such as the high-memory U7i Instances for large in-memory databases, the new Graviton4-powered R8g instances for demanding memory-intensive workloads, such as big data analytics, high-performance databases, and in-memory caches, with better price performance than any existing memory-optimized instance, and the Trainium2 purpose-built high-performance model training chipset for model training.
On reducing the model sizes front, AWS announced two relatively small models, the Titan Text Lite and Express models, supporting a wide range of text-related tasks, such as summarization, translation, conversational chatbot systems, and coding.
To alleviate storage data access limitation, AWS announced the new Amazon S3 Express One Zone high-performance storage class, which offers single-digit millisecond latency to support data-intensive applications, requiring thousands of parallel compute nodes to process data for use cases such as Generative AI model training. This announcement is the latest in a series of announcements improving storage systems' performance to scale to the Generative AI demand.
Generative AI’s popularity and adoption continue to grow, with over 60% of organizations with AI adoption reporting the use of Generative AI. This adoption will only be accelerated by advancements in model customization, Generative AI capabilities and computing, storage, and model sizes, resulting in further ubiquity with Generative AI making its way into smart, everyday devices and use cases.
Today, we see Generative AI capabilities in many tools, applications, and solutions that professionals use daily, such as Integrated Development Environments (IDEs) and Media Editing Software. This adoption emphasizes the necessity for organizations and individuals to acquire Generative AI proficiency and capabilities.
AWS went full force in incorporating Generative AI in existing tools and services and announced new Generative AI-powered tools this year, and some fun ones, such as Party Rock, which help customers build Generative AI applications quickly. Customers today build powerful tools on top of these services using their proprietary data and skillful workforce to create a sustainable competitive advantage.
The biggest announcement this year has been the preview availability of Amazon Q, a Generative AI-powered assistant capable of answering questions about structured and unstructured customer data and supporting developers and IT professionals in AWS consoles and the iOS Mobile App.
Q was first announced in Dec. 2020 as a feature of Amazon QuickSight, enabling customers to ask questions about their QuickSight-connected data in natural language. It became generally available on Sep 24, 2021. This year, Q became an independent offering with a diverse range of applications and use cases, including supporting IT professionals with building applications on AWS, researching best practices, and resolving errors, enhancing developer productivity with Amazon CodeCatalyst integration, supporting developers in modernizing their Java applications with Amazon Q Code Transformation, supporting contact center agents in Amazon Connect by recommending responses and actions, supporting Amazon Redshift developers in writing SQL queries and optimizing them, and augmenting Quicksight dashboard authoring activities, including building visuals and formatting them, creating complex calculations, and story-telling activities, including building formatted narratives, executive summaries, and the popular natural-language Q&A experience to help you get answers for questions beyond what is available in existing dashboards and reports.
The Generative AI adoption revolution is not restricted to Amazon Q. Many other functionalities have been added to existing services, such as Amazon Connect Contact lens and Customer Profiles features providing generative AI-powered post-contact summarization, and enabling contact center managers to more efficiently monitor and help improve contact quality and agent performance, and creating unified customer profiles, Amazon Transcribe Call Analytics Summarization feature, CodeWhisperer’s code security scanning and remediation, and Infrastructure as code (IaC) code assistant features, AWS Glue Studio notebook code companion for building data integration jobs, DataZone’s capabilities to automatically generate column and table names, and more detailed descriptions of the table and schemas, SageMaker’as well asanvas’s capability to explore and prepare data and deploy and use foundation models through its no-code experience, and Amazon CloudWatch logs and metrics natural language to querying.
AWS also announced AWS HealthScribe, a new service capable of generating clinical notes and analyzing patient-clinician conversations. HealthScribe provides several features, including turn-by-turn consultation transcripts with timestamps and speaker role identification, transcript and clinical note segmentation, summarization, and evidence mapping.
As Generative AI becomes increasingly ubiquitous, emphasis will be placed on improving backend operations, including improving its supporting services, integrating its entire ecosystem, and advancing observability, operations, and tools. These improvements are born out of the need to elevate Generative AI solutions to production-grade solutions, enabling development team collaborations, ease maintainability, and expedite time to market.
AWS has worked hard this year, making various platform advancements in vector stores, data integrations and governance, autonomous agents, and workflow orchestrations.
On the vector store front, AWS expanded support for vectors to RDS Aurora, which also saw a 20x performance improvement in Aurora-optimized vector reads, DocumentDB, OpenSearch Serverless, and DynamoDB via ZeroETL integration with OpenSearch, enabling customers to store source and vector data in the same database and eliminating the learning curve of learning new databases and APIs. They also announced that MemoryDB for Redis now supports vectors in preview, giving Generative AI applications an incredible single-digit millisecond latency with 99% information recall accuracy, providing unparalleled performance in vector databases. AWS also announced that Knowledge Bases now delivers a fully managed RAG experience in Amazon Bedrock with native integration to Pinecone, OpenSearch, and Redit Enterprise Cloud vector databases.
On the backend orchestration front, AWS announced the general availability of Amazon Bedrock Agents capable of executing complex tasks by dynamically breaking them down into a series of steps, creating an orchestration plan, and then carrying out the plan by invoking APIs and accessing relevant knowledge bases and providing a final response to the end user. This advancement also includes giving developers visibility to the Agent’s orchestration plan and the ability to modify it. Further, AWS announced a native integration of Amazon Bedrock with AWS Step Functions, enabling developers to directly invoke Bedrock’s CreateModelCustomizationJob and Invoke models APIs, as opposed to invoking a Lambda function to this end.
On the data integration front, AWS made a series of announcements, including
These integrations reduce the operational complexity of deriving insights from various data sources, breaking data silos, and managing multiple analytics tools, reducing costs and time to action.
They also announced several data store integrations with services, such as CloudTrail Lake integration with Amazon Athena, Amazon Connect contact center data integration with an analytics data lake, and Amazon Redshift integration for Apache Spark, with the latter making it easier to build and run Apache Spark applications on data from Amazon Redshift for analytics and ML.
AWS improved their Generative AI tooling, most notably the SageMaker Python SDK ModelBuilder and Schema Builder improvements. The first enables the conversion of models into SageMaker deployable models across ML frameworks and model servers, automatically selects compatible SageMaker containers, and captures dependencies from your development environment. The last helps manage serialization and deserialization tasks of model inputs and outputs. These tools also enable developers to deploy the model in their local environments for testing and experimentation and then deploy it on SageMaker with little effort. They also added a new inference capability to SageMaker, enabling the deployment of more than one model to the same SageMaker endpoint, optimizing deployment cost, and reducing latency.
Safety, Security, and Privacy are increasingly concerning to organizations and governments, and for a good reason. Generative AI’s disruptive power stands to introduce significant change in many aspects of our lives, including the way we work and, consequently, the economy. Governments and organizations are looking to set up appropriate guardrails around Generative AI and its supporting services to ensure data and infrastructure security, user privacy, and employee and model safety while winning the Generative AI against their competitors.
Accordingly, Safety, Security, and Privacy have gotten their fair share of attention this year, with AWS making several announcements in this space. The announcements come as a response to the market’s concerns.
AWS made a major announcement this year regarding model safety, announcing Guardrails for Amazon Bedrock. This feature enables developers to implement custom safeguards, denying topics and filtering harmful content from user interactions with applications. Amazon Bedrock models also got an upgrade, with Claude 2.1 reducing hallucinations by a factor of two compared to its predecessor and Titan Image Generator supporting invisible watermarks, ensuring the consumers of a generated image know it was machine-generated.
Earlier this year, AWS announced the general availability of AWS Clean Rooms a service that enables customers to share their data sources with others without sharing copies of their raw data. It works as an access control, privacy-preserving solution where data contributors dictate fine-grained access controls to their data, including query controls, query output restrictions, and query logging. For example, data contributors share a data catalog with their collaborators, specifying which columns can be retrieved via SELECT statements and which don't. At re:Invent this year, AWS announced AWS Clean Rooms Differential Privacy, a sophisticated method for preventing user identification via observing the difference in query results. It eliminates a small number of user records from the resulting sets to prevent identification while retraining result accuracy. They announced AWS Clean Rooms ML, an innovative approach to enabling customers to identify users within their collaborators' datasets with similar characteristics to theirs, enabling them to engage these users with offers, advertisements, and other meaningful ways. A customer can accomplish this by training a SageMaker lookalike model using their data and then sharing it with their collaborators. The collaborators, in turn, can run this model against their data to identify user records that look like the initial customer’s users.
The future of Generative AI appears promising and dynamic, marked by key trends shaping its trajectory. The future landscape of Generative AI is characterized by advancements in customization, model selection, enhanced capabilities, addressing limitations, widespread adoption, improvements in supporting services, and a growing emphasis on regulations and security, collectively playing a pivotal role in various domains.
AWS continues to be a driving force in shaping the future of technology, with 2023 being a testament to its innovation and agility. As we look towards 2024, the trends we see today will pave the way for a more integrated, efficient, and secure technological landscape underpinned by AWS’s continuous advancements. Stay tuned for more updates and insights as we navigate this exciting journey together.
1 This number is obtained by dividing the number of models hosted in Hugging Face model repository on Dec 11, 2023 by the number from Jan 6th in 2022. The latter is obtained using Way Back Machine: https://web.archive.org/web/20220106010152/https://huggingface.co/models
Dr. Khobaib Zaamout is the Principal Architect for AI Strategy at Caylent, where his main focus lies in AIML and Generative AI. He brings a solid background with over ten years of experience in software, Data, and AIML. Khobaib has earned a master's in Machine Learning and holds a doctorate in Data Science. His professional journey also involves extensive consulting, solutioning, and leadership roles. Based in Chestermere, Alberta, Canada, Khobaib enjoys a laid-back life. Outside of work, he likes cooking for his family and friends and finds relaxation in camping trips to the Rocky Mountains.
View Khobaib's articlesAhmed, currently a Senior Machine Learning Engineer at Caylent, plays a pivotal role in steering Gen AI projects to cater to the unique needs of clients. With a rich five-year tenure in the realm of machine learning, he has developed a deep expertise in designing and implementing holistic solutions in this domain. Prior to his tenure at Caylent, Ahmed dedicated a decade to the Oil and Gas sector, further enriching his profile with two years of consultancy work, focusing on the development and execution of bespoke solutions for diverse client needs. His academic journey is marked by a Master's degree in Computer Engineering with a specialization in Artificial Intelligence and Machine Learning from the University of Waterloo, Canada. This combination of practical industry experience and academic insight forms the cornerstone of his approach towards tackling intricate challenges in his field.
View Ahmed's articlesCaylent Catalysts™
Accelerate investment and mitigate risk when developing generative AI solutions.
Caylent Catalysts™
Learn how to improve customer experience and with custom chatbots powered by generative AI.
Caylent Catalysts™
Accelerate your generative AI initiatives with ideation sessions for use case prioritization, foundation model selection, and an assessment of your data landscape and organizational readiness.
Caylent Catalysts™
Educate your team on the generative AI technology landscape and common use cases, and collaborate with our experts to determine business cases that maximize value for your organization.
Read about the experiences our summer technology fellow had at Caylent, where she explored cloud computing, generative AI, web development, and more.
The AI industry is growing rapidly and a variety of models now exist to tackle different use cases. Amazon Bedrock provides access to diverse AI models, seamless AWS integration, and robust security, making it a top choice for businesses who want to pursue innovation without vendor lock-in.
ARIA is the World's First Generative AI-Powered Virtual Building Assistant Designed to Enhance Building Efficiency and Reduce Greenhouse Gas Emissions